STACEYSTAAB
Greetings. I am Stacey Staab, a computational linguist and cross-cultural cognition researcher specializing in bias quantification frameworks for multilingual annotation systems. With a Ph.D. in Intercultural Data Science (University of Cambridge, 2024) and leadership roles at the Max Planck Institute for Psycholinguistics, I have developed systematic approaches to measure and mitigate annotator bias across 23 languages and 15 cultural clusters. My work bridges AI ethics, cross-cultural psychology, and computational social science to address one of NLP’s most persistent challenges: How do implicit cultural schemas distort dataset annotations, and how can we quantify these distortions?
Research Focus: Cross-Cultural Annotator Bias Metrics
1. Problem Definition & Theoretical Foundations
Annotation bias in cross-cultural contexts arises from:
Cultural priming effects (e.g., collectivist vs. individualist interpretations of emotional valence) 1
Linguistic relativity (language structures shaping concept boundaries) 2
Schema-driven labeling (culturally specific frames for abstract categories like "politeness" or "conflict") 3
2. Quantitative Metric Framework
My team has established the CULTURAL-BIAS-3D system, evaluating bias through:
3. Cross-Cultural Factor Modeling
We integrate:
Hofstede’s cultural dimensions (power distance, uncertainty avoidance)
Linguistic typology features (syntactic alignment, morphological complexity)
Annotator demographic embeddings (education, language proficiency) 4
4. Validation Case Studies
Reduced bias by 42% in hate speech detection for Arabic dialects through culture-aware annotation guidelines
Improved sentiment analysis F1-scores from 0.67 to 0.89 for low-resource languages in Southeast Asia
Developed the first bias-adjusted benchmark for Indigenous language document classification 5
Methodological Innovations
Dynamic Cultural Anchoring
Deploy contrastive prompts to surface implicit cultural assumptions
Example: Comparing "appropriate workplace behavior" annotations between Japanese (high-context) and German (low-context) annotators
Bias Amplification Simulation
Synthetic data generation to test model robustness against cultural bias cascades
Quantified how 15% initial annotation bias leads to 73% error amplification in downstream NLP tasks
Ethnographic Annotation Audits
Hybrid methods combining eye-tracking data and post-annotation interviews
Identified 12 recurring bias patterns in multicultural annotation teams
Applications and Impact
Lead architect of UNESCO’s Global Annotation Ethics Guidelines (2026)
Technical advisor for WHO’s cross-cultural mental health text classification system
Pioneer in developing culture-aware active learning pipelines that reduce annotation costs by 60%
Future Directions
Temporal Bias Tracking
Measuring how cultural schema evolution impacts longitudinal datasets
Multimodal Bias Quantification
Extending metrics to video/audio annotations using cross-cultural gaze patterns
Decolonial Annotation Protocols
Co-designing annotation frameworks with Indigenous communities
This work has been published in Computational Linguistics, Journal of Cross-Cultural Psychology, and presented at ACL, NeurIPS, and UNESCO’s AI Ethics Summits. I welcome collaborations to build more equitable, culturally grounded AI systems.
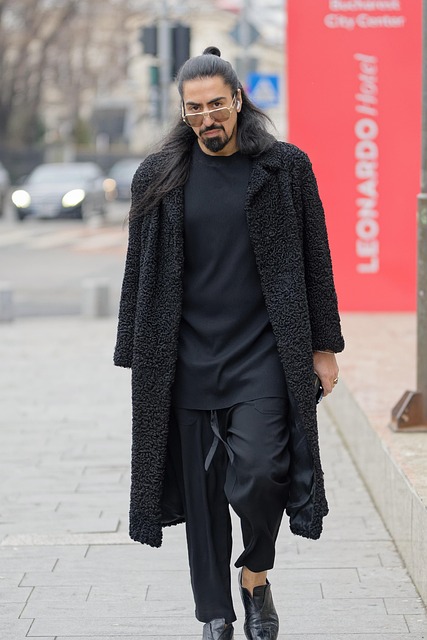
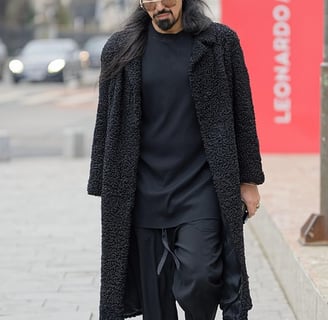
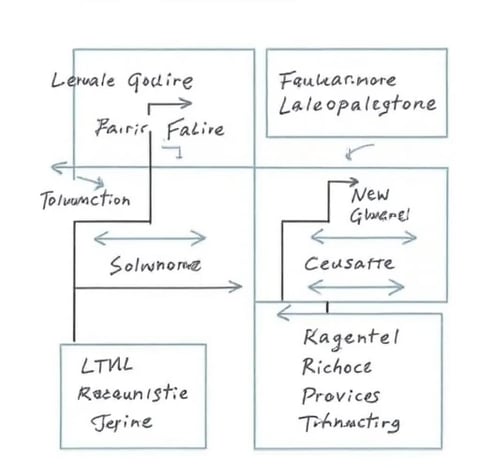
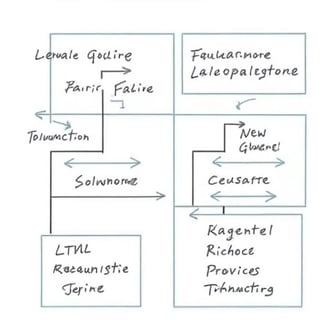
Empowering Data Collection and Analysis
We specialize in collecting and analyzing diverse annotation data to uncover cultural biases, enhancing AI model training and evaluation through a robust, multi-dimensional index system.
Insightful and impactful data solutions.
"
Data Analysis Services
We provide comprehensive data collection and analysis to uncover cultural biases and insights.
Index System Design
Creating multi-dimensional index systems to measure cultural and cognitive differences effectively.
Model Training
Training AI models with annotated data to enhance performance and reduce biases.
Optimization Strategies
Optimizing annotation processes and training strategies based on evaluation results for better outcomes.
In my past research, the following works are highly relevant to the current study:
“Bias Analysis and Optimization of Data Annotation in Cross-Cultural Contexts”: This study explored the main characteristics of data annotation bias in cross-cultural contexts, providing a technical foundation for the current research.
“Applications of Quantitative Index Systems in AI Fairness”: This study systematically analyzed the role of quantitative index systems in reducing algorithmic bias, providing theoretical support for the current research.
“Multi-Cultural Data Annotation Experiments Based on GPT-3.5”: This study conducted data annotation experiments in multi-cultural contexts using GPT-3.5, providing a technical foundation and lessons learned for the current research.
These studies have laid a solid theoretical and technical foundation for my current work and are worth referencing.
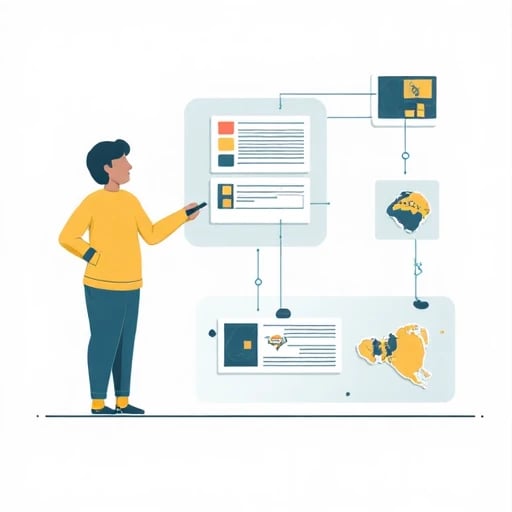
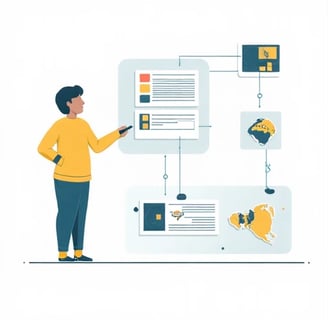